Concepts of health and disease as a barrier to progress
This essay was the runner-up in the Homeworld Ideas writing contest. To read it on the Homeworld website, click here.
We tend to think of health in discrete terms. Either you’re healthy, or you’re sick. Similarly, we put diseases into neat-seeming categories: cancer, arthritis, epilepsy, hypertension, psoriasis, and so on. Yet, our categorical frameworks and language — how we think and talk about disease — are mental shortcuts that may actually be holding us back.
We create categories because we find them useful for decision making. Minds, like metal dies, make sense of reality’s raw extrudate by shaping it into definite forms. Yet this sensemaking process can distort the underlying truth. When it comes to our health, there is an arbitrariness to how we categorise disease that perhaps says more about the evolution of our medical systems and specialties than it does about our biology.
Medical specialties as we know them today emerged in the early-to-mid 1800s1. The impetus to master complex high-volume procedures, improve outcomes, and better compete for patients supplied the initial incentives to specialise. Ophthalmology, for instance, was an early breakaway; the first eye hospital, Moorfields, was founded in London in 18052. By centralising patients, eye hospitals enabled early specialists to gain sufficient volume of practice to reliably perform the intricate, yet effective, surgery required to remove cataracts and foreign objects in the eye3.
In many cases, operational pragmatism drove the functional specialisation of medical institutions. The spread of ‘lunatic asylums’, intended to sequester the mentally ill away from the general sick population, facilitated the relatively early emergence of psychiatry. Funding for specialised institutions, like Germany’s Bethel epilepsy centre, often came from philanthropic donors with the explicit aim of treating underserved patient populations. It was natural for doctors working at such functionally specialised institutions to adopt a specialty.
Post-revolutionary France was the first country to adopt a broad system of medical specialisation, starting from the 1840s. As the centre of the Enlightenment, Parisian institutions were predisposed to allow reductionist ideas to first creep into medical practice and take hold. In his history of medical specialisation4, Dr. George Rosen suggested that:
“Specialisation was not a consequence of the accumulation of knowledge, but rather of a new conception of disease: it was specifically the influence of localist pathological thinking, based on pathological anatomy and subsequently on new technologies like the ophthalmoscope and laryngoscope, that created ‘foci of interest’ in organ systems around which specialties could develop.”
Specialisation provided a means to distinguish oneself in the Parisian institutional world, which, at the time, placed great emphasis on the advancement of scientific knowledge. By specialising, physicians increased their chance of remaining at the forefront of knowledge within their chosen domain.
Although Paris may have been the nucleus, specialisation spread rapidly outwards: first to Vienna, then to other European cities, and, by the 1860s, to North America. While growth was slow at first, the proportion of medical specialists exploded at the turn of the 1900s. In 1845, about 10% of Parisian physicians identified as specialists (excluding surgeons); by 1905, that share had hit 35% in Paris, and 30% in Berlin1. This is not to say there was no resistance to the increasing specialisation of medicine and the rise of ‘special hospitals’. Britain, in particular, was slow to specialise at scale; its leading doctors expressed concerns over wasteful duplication of resources and feared that the loss of a broader perspective would harm patients5. As the surgeon Sir Benjamin Brodie put it in 1860:
“Diseases generally are so connected with each other, and a knowledge of one is so necessary to a right understanding of another, that no one who limits his attention to any given disease, can be so competent to investigate its nature, and to improve its method of treating it, as those are who have a wider field of observation, and who are better acquainted with general pathology.”
Yet, specialisation won. Today, two-thirds of doctors in the OECD countries are specialists. The long list of medical specialties is an eclectic collection of domains that are organ-based (hepatology, nephrology, neurology…), disease or symptom-based (oncology, allergy, pain medicine…), and procedure or technique-based (anaesthesiology, pathology, radiology…). Nobody would design such a Byzantine6 system from first principles. Rather, the specialties developed organically from contingencies grounded in the practical realities of medical practice, less so the biological realities.
Molecular biology, pathophysiology, and pharmacology grew up in medicine’s shadow, so naturally they inherited its concepts. This inherited framing has trickled down to how we think about diseases and their treatments more broadly. Consider psoriasis, uveitis, Crohn’s disease, and rheumatoid arthritis. Biologically, these are all complex inflammatory autoimmune diseases which share similar causes and treatments; for instance, all can be effectively treated by a class of drugs that block the pro-inflammatory TNF protein. Despite their similar biology, these conditions are managed by different specialties. In another world, they might all be managed by an inflammation specialist who sees them as manifestations of the same underlying TNF-dysregulation disease.
While current categorisations of disease are not invalid — they are, after all, functional — questioning the inherent assumptions within them may help lay the groundwork for a new way of conceptualising, and treating, illness.
When we create categories we implicitly assume that meaningful boundaries can be drawn between illness and health, and between diseases. Take the debate over prediabetes, which the American Diabetes Association defines as an A1C of 5.7% to 6.4% (where A1C is a measure of blood sugar levels). This threshold is arbitrary — other agencies like the WHO use different thresholds which shift the estimated prevalence by millions7. While pre-diabetics are at heightened risk of progressing to diabetes, the risk is low, on the order of a few percent per year8. Regression to normal blood sugar levels is about as common as progression to diabetes.
This challenge of drawing thresholds exists within diseases too, even among diseases with relatively well-defined genetics. Mutations in the sodium channel protein SCN1A lead to a spectrum of epilepsies that range from relatively mild and self-resolving to the frequent, disabling seizures of Dravet syndrome. Yet, the phenotype of Dravet syndrome is itself highly variable. There is no clean solution to divide up the SCN1A epilepsies; any subdivision contains flaws. Age, response to prior treatment, and seizure type variability further stymie classification. While seizure severity is correlated with the magnitude of structural mutations in SCN1A9, classification on the basis of genetics is no easy solution. Family members with the same genetic mutation can have widely differing expressions — one child may be disabled by daily seizures, while the other is spared10.
Similar issues are seen in psychiatry too: there is substantial genetic overlap and shared risk factors among attention deficit hyperactivity disorder, bipolar disorder, major depressive disorder, and schizophrenia11. What separates someone who suffers from one psychiatric disease from another, or determines whether someone is considered mentally healthy or unwell, might be a particular quirk of their environment or life trajectory — for example the season in which they were born, or whether they grew up in an urban environment12.
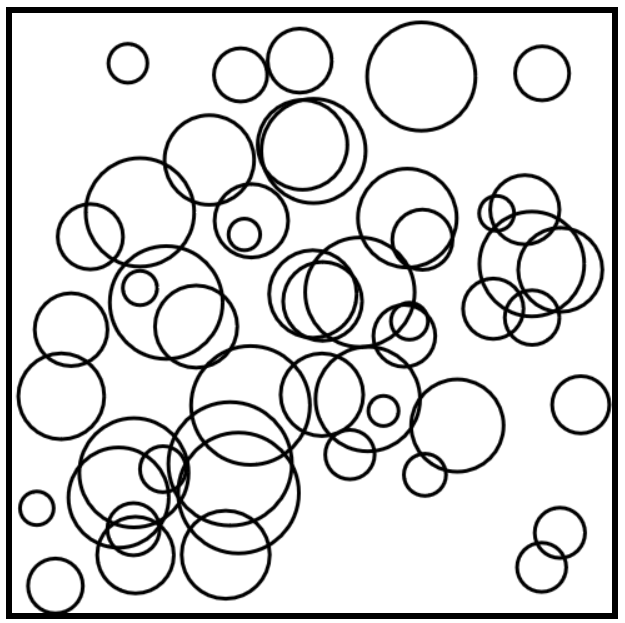
We break diseases into categories to help us understand and treat them, but we must not forget that a category is not real — it’s a label. Although categories, thresholds, and boundaries may be artificial, they have real-world consequences. Categories can be useful abstractions. But it’s worth asking: useful for whom?
When you must allocate limited resources and decide who can and should visit the hospital, it makes sense to draw a distinction between healthy and sick. When you must route people to the appropriate specialty, prescribe drugs with a specific label, and assign an ICD code to bill them, it makes sense to argue over the terminology for their specific affliction.
When motivations beyond the accurate representation of underlying biology creep into the categorisation process, distortions are introduced. To see why, we can think of categorising a disease as akin to the mathematical technique of ‘dimensionality reduction’. This is the process of projecting a multi-dimensional object or dataset onto a lower dimensional mapping — like how light thrown onto a three-dimensional shadow puppet casts a two-dimensional shadow. Once data has been projected onto a lower dimension, you can then draw boundaries around the individual shapes that emerge, and give them separate names.
Throwing away dimensions is a useful trick because it allows us to focus on the variables (dimensions) that we deem most important, while retaining as much information as possible. If you’ve reduced the dimensions cleverly, then the lower-dimensional representation should still look much like the true shape. Imagine twisting a shadow puppet around in a stable beam of light: the puppet’s three-dimensional shape doesn’t change, but its shadow does. The more the puppet is twisted away from its intended orientation, the less faithfully its shadow represents its higher-dimensional form.
One of the risks that comes with dimensionality reduction is loss of relevant information and distortions of the truth. Depending on how you collapse the dimensions, separate entities can look like the same thing: think of two shadow puppets, one obscured behind the other. Performing a dimensionality reduction means making trade-offs; some information must be discarded. When we created our taxonomy of disease we cast off some information to make room for human constructs and conveniences — like specialties — which necessarily leaves less space to better represent the underlying biology. Biology has been called “the science of exceptions”, but it only seems this way because nature likes to make shapes that don’t fit neatly into low-dimensional boxes.
The modern concept of precision medicine — tailoring therapeutic interventions to an individual’s particular biology or characteristics — is an attempt to address the problem of over-broad disease categories by adding back missing dimensions. Even so, our current approach is trapped within the gravity well of pre-existing constructs. When you talk in terms like ‘a precision medicine based approach to psoriasis’, you are already operating within the existing framework. Enlightenment Paris casts a long shadow.
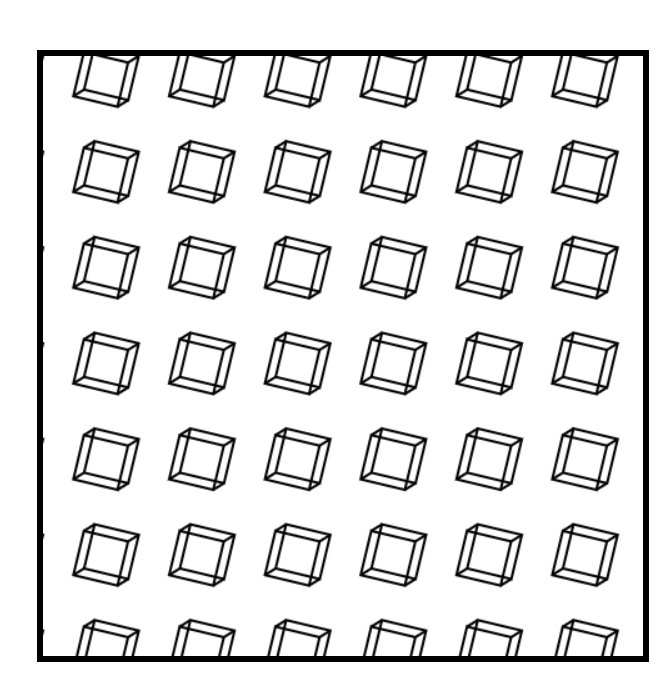
What if we were to build precision medicine from scratch? How might we unravel the fan of dimensionality as far as possible, and approximate nature as she really is?
Imagine for a moment you were in some idealised world without computational or instrumental limits. First, you might map all the dimensions along which our biology could vary. Then, having done that, you could plot the values associated with good health on this multidimensional chart.
This is a way of thinking of diseases not as specific entities, but as mappings to regions in a continuous ‘health landscape’ or ‘phase space’.
Discrete ways of thinking beget discrete solutions. Like how you might repair a car: diagnose the problem, apply a fix, or replace the part. This is no way to cure a complex, adaptive, systemic condition like cancer — certain types with simple molecular drivers, yes — but others must be gradually coaxed out of existence.
People are not a collection of individual symptoms, diseases, or parts. We are dynamic, adaptive systems where genetics, risk factors, comorbidities, and disease processes intermingle into a holistic state unique to each individual. Thinking about health and disease as a continuum engenders a way of thinking that is fluid and systemic — if you lower the mental walls that categories erect, it seems easier to move between them.
Dynamism is more natural when we think in continuums instead of rigid categories. Within such a framework, we can conceptualise a disease risk factor as a force pushing an organism’s state away from a healthy position — like a boat adrift on a calm sea being pushed out into stormy waters. Certain genetic mutations (like the CFTR mutations that cause cystic fibrosis) would be particularly strong directional currents. This implies the current state of the system is not sufficient to describe its health, we also need the rate and direction at which it changes13. It’s easy to forget about path-dependence when we see the world as static slices.
To treat, or prevent, disease we would have to apply a countervailing force with similar magnitude to push the system back to a desirable position. The further from health, the harder it is to get back. The greater, or longer, the force that would need to be applied. Intervening in the system changes it, so we would have to continually measure and adjust the force and direction of intervention. Drugs are one way of applying this countervailing force, as is exercise, nutrition, and other interventions.
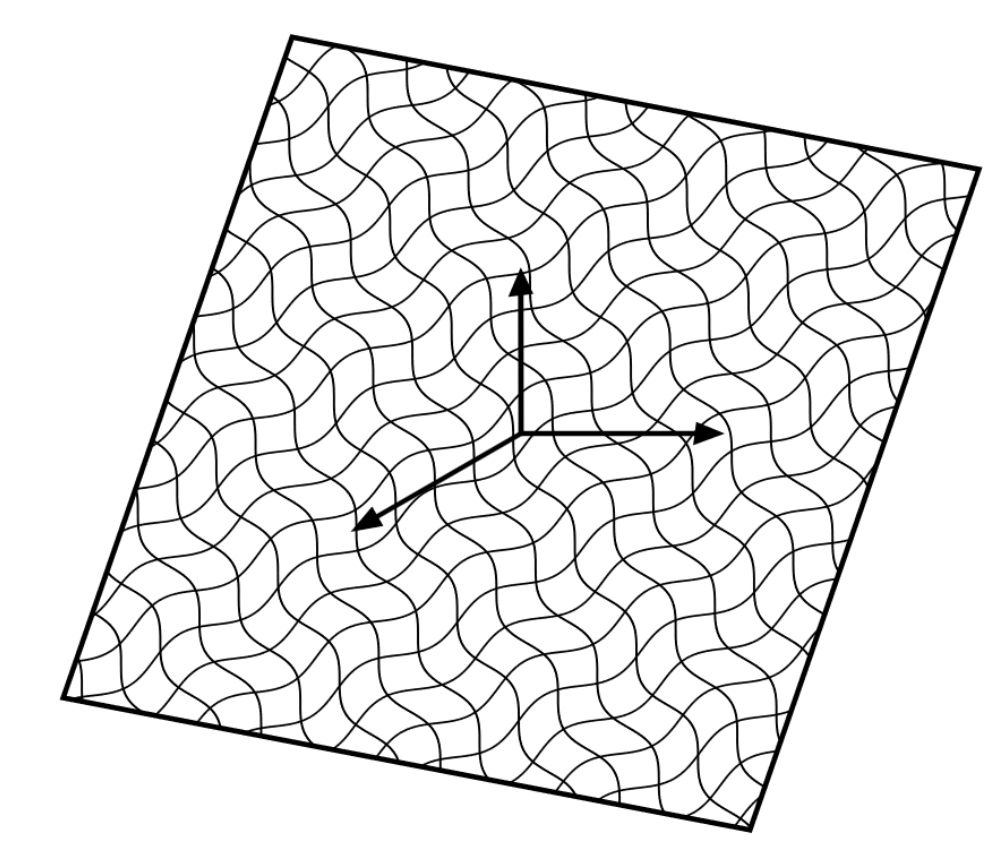
We could even take a homeostatic perspective and think of health not as a region of the landscape, but as a measure of system responsiveness; the ability to resist forces that might push a system out of equilibrium. Some have made similar suggestions that ageing is akin to a loss of system ‘springiness’. The net effect of living being the slow accumulation of drift.
What I like about this view of disease is that it shows how much more room remains for biotech innovation. All the drugs the biopharmaceutical industry has produced until now account for only a tiny fraction of the ways we could perturb our biology; each a single transformation from point A to point B on an infinite landscape.
In the future, your annual (or more frequent) medical check-up might track thousands, or millions of parameters. Your doctor could estimate the rate and direction of drift from one check-up to the other, and prescribe a tailored mix of interventions (drugs, exercise, nutrition, etc.) to counteract the net forces pushing away from health — perhaps with the help of a predictive artificial intelligence (AI) model. We wouldn’t necessarily find it useful to assign disease labels in such a world, instead we might rely on exquisitely detailed models that represent our health states as mathematical objects.
To get to this future, we’ll need to overcome the practical limits to this ever more precise description. Every cut we take to define a population more precisely shrinks the size of that population. If we don’t change our approach, there will eventually be an economic limit to precision medicine. Taken to its extreme, we cannot run eight billion n-of-1 trials. To overcome this practical and epistemological barrier we’ll need to train AI models to predict how our health interventions will modulate out-of-sample states, and then validate those predictions. We’ll need to find a balance between high-dimensional representations that capture as much biology as possible, while remaining tractable to measure.
This vision will take decades to achieve in full, of course, but that doesn’t mean we can’t start laying the groundwork. We can engineer better imaging and measurement instruments to more precisely capture and measure biological states, and critically, the rate and direction of how they are changing. We can start testing our expanding library of approved drugs — alone and in combination — to see how they affect biological systems along as many dimensions as we can measure, and characterise their ‘intervention vectors’. These perturbation maps could be built up in ever greater detail: starting with cells, to tissue and organs, then full bodies. We can work with regulators to further explore disease-agnostic indications, and develop and validate new AI-discovered biomarkers for clinical trials.
Perhaps most of all, we’ll need to be open to new ways of reasoning about biology and disease: if we hope to cure all disease, we may eventually have to abandon the concept.
-
Weisz, George. “The emergence of medical specialization in the nineteenth century.” Bulletin of the history of medicine vol. 77,3 (2003) ↩ ↩2
-
Dr. George Rosen’s book is ‘The Specialization of Medicine with Particular Reference to Ophthalmology’. The quote is taken from George Weisz’s summary of Rosen’s work in reference #1 ↩
-
Even though public hospitals probably did originate in the Byzantine empire ↩
-
For a review of the epidemiology of pre-diabetes and the effect of the different thresholds on estimated prevalence, see this paper ↩
-
van Herpt, Thijs T W et al. “Lifetime risk to progress from pre-diabetes to type 2 diabetes among women and men: comparison between American Diabetes Association and World Health Organization diagnostic criteria.” BMJ open diabetes research & care vol. 8,2 (2020) ↩
-
Zuberi, S M et al. “Genotype-phenotype associations in SCN1A-related epilepsies.” Neurology vol. 76,7 (2011) ↩
-
Miller IO, Sotero de Menezes MA. “SCN1A Seizure Disorders” GeneReviews (2007) ↩
-
Brainstorm Consortium et al. “Analysis of shared heritability in common disorders of the brain.” Science (New York, N.Y.) vol. 360,6395 (2018) ↩
-
Robinson, Natassia, and Sarah E Bergen. “Environmental Risk Factors for Schizophrenia and Bipolar Disorder and Their Relationship to Genetic Risk: Current Knowledge and Future Directions.” Frontiers in genetics vol. 12 686666. 28 Jun. 2021 ↩
-
Conceptually, a bit like the first derivative of the position on the health phase space ↩